The understanding of proteins, particularly those involved in diseases like cancer and COVID-19, relies on identifying their chemical structure and determining their binding partners. However, this task poses a significant challenge.
“The search space for proteins is enormous,” explained Brian Coventry, a research scientist at the Institute for Protein Design, University of Washington and The Howard Hughes Medical Institute.
Typically, a protein consists of 65 amino acids, and with 20 different amino acid choices at each position, the number of potential binding combinations is greater than the estimated number of atoms in the universe.
Coventry and his team published a study in May 2023 in the journal Nature Communications, where they utilized deep learning methods to enhance computational protein design. This approach resulted in a 10-fold increase in success rates for binding designed proteins to their target proteins.
According to study co-author Nathaniel Bennett, a post-doctoral scholar at the Institute for Protein Design, incorporating deep learning methods provided significant improvements by evaluating the quality of the interfaces where hydrogen bonds form or from hydrophobic interactions, rather than exhaustively calculating individual energies.
Deep learning, a form of computer analysis based on recognizing patterns in data, was used to learn and transform protein sequences and structures rapidly, leading to highly accurate models.
The authors employed deep learning-based tools such as AlphaFold 2 and RoseTTA fold, developed by the Institute for Protein Design, to augment their de novo protein binder design protocol.
To address the vast computational requirements of their study, the authors utilized the Frontera supercomputer, which enabled parallelization of the protein design trajectories. This approach significantly accelerated the research process.
While the results of the study showed promising progress in increasing the success rate for designed proteins to bind to their target proteins, Coventry emphasized that there is still room for improvement. The next challenge involves tackling even more complex targets like viruses and cancer T-cell receptors.
Coventry highlighted the need for further optimization of software tools and increased computational power to advance protein design for developing cancer-fighting drugs and other life-saving treatments.
The study conducted by Coventry and his team represents a significant step forward in computational protein design. The integration of deep learning methods and the utilization of advanced supercomputing resources have paved the way for improved drug development and personalized medicine.
More information: Nathaniel R. Bennett et al, Improving de novo protein binder design with deep learning, Nature Communications (2023). DOI: 10.1038/s41467-023-38328-5
Citation:
Deep learning for new protein design (2023, August 3)
retrieved 3 August 2023
from https://phys.org/news/2023-08-deep-protein.html
This document is subject to copyright. Apart from any fair dealing for the purpose of private study or research, no
part may be reproduced without the written permission. The content is provided for information purposes only.
Denial of responsibility! SamacharCentrl is an automatic aggregator of Global media. In each content, the hyperlink to the primary source is specified. All trademarks belong to their rightful owners, and all materials to their authors. For any complaint, please reach us at – [email protected]. We will take necessary action within 24 hours.
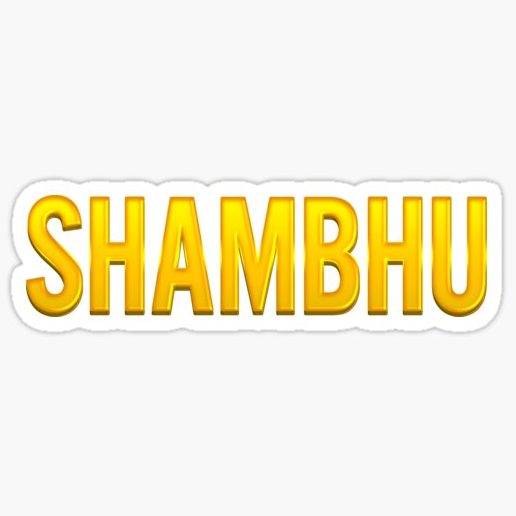
Shambhu Kumar is a science communicator, making complex scientific topics accessible to all. His articles explore breakthroughs in various scientific disciplines, from space exploration to cutting-edge research.