Participants and data
This is a natural history, non-interventional study of cognitively normal individuals ages 35–65 years, carrying risk factors for late-onset AD such as a family history and/or APOE4 genotype. Participants were recruited at the Weill Cornell Medicine (WCM) Alzheimer’s Prevention Program between 2018 and 2023 by self-referral, flyers, and word of mouth.
Our inclusion and exclusion criteria were previously described40,41,42,43. Briefly, all participants had Montreal Cognitive Assessment (MoCA) score ≥ 26 and normal cognitive test performance by age and education40,41,42,43. Exclusion criteria included medical conditions that may affect brain structure or function (e.g., stroke, any neurodegenerative diseases, major psychiatric disorders, hydrocephalus, demyelinating disorders such as Multiple Sclerosis, intracranial mass, and infarcts on MRI), use of psychoactive medications, and contraindications to MR or PET imaging. None of the participants reported using glucocorticoid medications. All participants received medical, neurological, laboratory, cognitive exams, volumetric MRI and 31P-MRS scans within 6 months of each other. Approximately half of our participants also receive PET imaging. A family history of late-onset AD was elicited using standardized questionnaires40,41,42,43. APOE4 genotype was determined using standard qPCR procedures. Participants carrying one or two copies of the APOE4 allele were grouped together as APOE4 carriers (APOE4+) and compared to non-carriers (APOE4−).
The patients’ sex was determined by self-report. Our study protocol involves a 1:3 enrollment ratio of men to women, with approximately equal representation of premenopausal, perimenopausal, and postmenopausal statuses among women (1:1:1 ratio). Determination of menopausal status was based on the Stages of Reproductive Aging Workshop (STRAW) criteria49 with hormone assessments as supportive criteria44. Participants were classified as premenopausal (regular cycler), perimenopausal (irregular cyclers with interval of amenorrhea ≥ 60 days or ≥ 2 skipped cycles) and postmenopausal (no cycle for ≥ 12 months)44. Information on hysterectomy/oophorectomy status and hormone therapy (HT) usage was obtained through review of medical history.
Standard protocol approvals, registrations, and patient consents
All methods were carried out in accordance with relevant guidelines and regulations. All assessments and imaging procedures were approved by the Weill Cornell Medicine Institutional Review Board and Radioactive Drug Research Committee. Written informed consent was obtained from all participants.
Cortisol assessment
Participants received a blood draw by venipuncture between 8 and 9am after an overnight fast. Blood samples were centrifuged and shipped overnight to CLIA-certified Boston Heart Diagnostics (Framingham, MA). Serum cortisol concentration (µg/dL, micrograms per deciliter) was measured with Electrochemiluminescence Immunoassay (ECLIA) on a Roche Cobas e601/e602 analytical unit for immunoassay tests using Electrochemiluminescence technology (ECL) [Roche Diagnostics; Basel, Switzerland], with intra-assay coefficients of variation ranging from 1.8 to 7.1% for high concentrations and low concentrations. The limits of detection of the test were 0.054–63.4 μg/dL.
Cognitive measures
Participants underwent a cognitive testing battery40,41,42,43, including Rey Auditory Verbal Learning Test (RAVLT) immediate and delayed recall, Wechsler Memory Scale Logical Memory immediate and delayed recall, Trail Making Test (TMT) B, FAS, animal naming, and Boston object naming. Higher scores across all cognitive endpoints indicate better performance, except for TMT, for which higher scores indicate slower task completion. Cognitive scores were standardized prior to analysis.
Brain imaging
Image acquisition
All participants received a 3D volumetric T1-weighted MRI scan on a 3.0 T GE MR 750 Discovery scanner (General Electric, Waukesha, WI) [BRAVO; 1 × 1 × 1 mm resolution, 8.2 ms repetition time (TR), 3.2 ms echo time (TE), 12° flip angle, 25.6 cm field of view (FOV), 256 × 256 matrix with ARC acceleration] using a 32-channel head coil, using published protocols40,41,42,43. PET scans were acquired on a Siemens BioGraph mCT 64-slice PET/CT operating in 3D mode [70 cm transverse FOV, 16.2 cm axial FOV] following standardized procedures40,41,42,43. Summed images were obtained 40–60 min post-injection of 5 mCi of 18F-fluoro-deoxyglucose (FDG), and 60–90 min post-injection of 15 mCi of 11C-Pittsburgh Compound B (PiB). All images were corrected for attenuation, scatter and decay.
The 31P-MRS scan was acquired on the same scanner as the MRI, typically on the same day, using a dual tuned 32-channel 31P/1H quadrature head coil (Clinical MR Solutions, Brookfield, WI) [2048 points, 5000 Hz sweep width, 2000 ms TR, 2 averages, 55° flip angle at 51.3 MHz, 24 cm FOV]36,37,42. A 3 Plane Localizer image with 20 images in each orthogonal direction was acquired. Shimming was performed using a 1H single voxel technique placed over the entire brain. A high-resolution, 8-slice sagittal T2-Fluid Attenuated Inversion Recovery sequence [FLAIR; 2200 ms TR, 12 ms TE, 780 ms inversion time (TI), 24 cm FOV, 0.94 × 0.94 mm] was acquired with a 5 mm slice thickness at the same location as each of the 31P-MRS CSI slices for reference. MRS data were processed using XSOS written in IDL36,37,42. Raw data was processed using Hamming and Fermi k-space filters, 20 Hertz exponential filtering and zero-filling in time, x and y-domains prior to 3D Fast Fourier Transformation. This resulted in a 16 × 16 image of 1.5 × 1.5 × 3.0 cm voxels with the signal intensity in each voxel corresponding to the peak area of the 31P metabolite. Peak area integration was performed around each of four well-resolved resonance peaks: phosphocreatine (PCr), α-ATP, β-ATP and γ-ATP moieties. The PCr peak was set at 0.0 ppm and susceptibility corrections performed by an experienced analyst (JPD). The ratio of PCr over total ATP (sum of α-, β- and γ-ATP) was computed.
Multiparametric image analysis
The 3D T1-weighted BRAVO MRI scans were first processed in Statistical Parametric Mapping (SPM8)50 implemented in Matlab 2021 (MathWorks; Natick, MA). For each participant, we used the Normalized Mutual Information routine of SPM850 to align the T1 BRAVO sequence to each PET scan, and to the reference T2-FLAIR acquired at exactly the same location as the 31P-MRS CSI slices. The parametric metabolite MRS maps were then aligned with the skull stripped 3D T1-weighted FreeSurfer scan. Volumetric MRI scans were resampled to a 256 × 256 × 256 matrix array whereas the parametric metabolite MRS maps were resized to 256 × 256 images but not interpolated beyond the original 16 × 16 × 8 matrix given partial volume errors would occur. The co-registered images were quantified using the subcortical gray and white matter segmentation tools implemented in FreeSurfer 6.051 running under the Centos 7 Linux environment and Desikan-Killiany Atlas-based regions of interest (ROI)52 applied to the aligned MRI for regional sampling.
We focused on ROIs with known AD vulnerability31: medial temporal lobe (hippocampus, amygdala, entorhinal and parahippocampal gyrus), middle and superior frontal gyrus; posterior cingulate gyrus and precuneus; inferior, middle and superior temporal gyrus; and inferior and superior parietal gyrus. Hippocampus was examined in analysis of MRI volume, whereas the medial temporal ROI was used for MRS and FDG-PET due to their lower resolution. For PiB analysis, we created an AD-mask by averaging parietal, temporal, frontal, posterior cingulate and precuneus ROIs. Biomarker data were normalized by modality-specific confounders: MRI volumes were adjusted by total intracranial volume; 31P-MRS ATP measures were normalized to PCr; FDG- and PiB-PET measures were normalized to cerebellar gray matter uptake obtained with FreeSurfer to derive standardized uptake value ratios (SUVR).
Clinical covariates
In Model 1, all analyses were adjusted by age, sex, and APOE-4 status. Cognitive analyses were also adjusted by years of education. In Model 2, we further examined midlife health variables, including smoking (self-reported as ever vs. never smoker), hypertension (systolic BP ≥ 140 mmHg, a diastolic BP ≥ 90 mm Hg, or self‐reported antihypertensive medication use), hypercholesterolimia (total cholesterol ≥ 240 mg/dL or self-reported use of cholesterol-lowering medications), diabetes (fasting glucose level ≥ 126 mg/dL or self‐reported use of glucose-lowering medications), and depression (Hamilton test and/or the Patient-Reported Outcomes Measurement Information System (PROMIS) Depression measure or self-reported use of antidepressant medications). For women, we also examined menopausal status (postmenopausal vs. pre/perimenopausal) and HT use (user vs. never-user).
Statistical analysis
Analyses were performed in R v.4.2.0 and SPSS version 28. Clinical measures were examined using general linear models or chi-squared tests, as appropriate. As cortisol and PiB SUVR measures did not follow a Gaussian distribution, we used the bestNormalize R package to identify the optimal normalization transformation for each measure. The standardized asinh(x) transformation was selected for cortisol and AD-mask SUVR, and the OrderNorm transformation for regional PiB SUVR values. Following the transformations, all variables passed the Shapiro Wilks test for normality and their histograms displayed normal distributions.
Our primary outcome for PiB-PET was Aβ load in AD-mask. For the other modalities, we used Principal component analysis (PCA)53 to reduce the dimensionality from the original 10 ROIs across each imaging modality, using varimax rotation with kaiser normalization. An eigenvalue threshold of 1.0 was predetermined to select factors for inclusion in hypothesis testing. PCA extracted distinct factors for each modality: two factors from MRI gray matter volumes, two factors from PCr/ATP, three factors from CMRglc. The composition of the extracted factors is detailed in Supplementary Table 1. These factors accounted for > 68% of the total variance in the biomarker panel for each modality. For all participants, we computed standardized scores for each identified factor, which represent a linear combination of the regional biomarker measures showing substantial loading (coefficients > 0.55) within each respective factor. Our primary analysis focuses on PCA-derived factors, as this approach effectively captures the interrelatedness of regional biomarker data and streamlines the hypothesis testing process. For comparison with previous studies23, we also included total brain volume.
Multivariable linear regression models were trained to test for associations between cortisol and each biomarker and cognitive outcome, adjusting by covariates. We then examined sex modification effects by developing linear regression models including multiplicative interaction terms, for all outcomes. Each model contained cortisol, sex, and their interaction as covariates, as well as clinical covariates. Estimates are presented for the overall study sample as well as by sex. The stratified analysis was performed to investigate hypothesized differences in the strength of the correlations by sex and to mitigate the effects of its confounding on the overall correlations. Analyses were performed separately for each biomarker outcome. Results were considered significant at P < 0.05. Given that each biomarker was examined independently and the PCA method effectively reduced the dimensionality of our data, correction for multiple comparisons was not performed.
Sensitivity analysis
Associations of cortisol and regional biomarker measures
To examine which regions were involved in the above effects, multivariable linear regressions were used to test for associations between cortisol and sex, and their interactions, in the full ROI panel. Analyses were performed separately for each biomarker outcome. Results are reported at P < 0.05. To address the issue of multiple comparisons, we applied the Benjamini–Hochberg correction method, which controls the false discovery rate (FDR) by adjusting the significance threshold to account for intercorrelated measures54.
Effects of menopausal status
We used multiple linear regressions to test for effects of menopausal status on the associations of cortisol and biomarker outcomes. To reduce the number of comparisons and therefore the potential for Type I error, this analysis was limited to biomarker measures demonstrating significant main effects of sex in their relationship with cortisol levels. As shown in Supplementary Table 4, the postmenopausal group was older than the premenopausal group, while no age difference was found between postmenopausal and perimenopausal groups, or compared to men. Given these considerations and the narrower biomarker range in the premenopausal group, analysis was restricted to age-comparable groups (postmenopausal, perimenopausal, and men). In combination with age correction procedures, this approach ensures a more accurate examination of menopausal status effects. We structured regression models to explore pairwise combinations between groups (postmenopause vs. men; perimenopause vs. men), with each model containing cortisol, sex, and their interaction, and clinical covariates. Results were considered significant at P < 0.05.
Mediation analysis
We used multiple linear regressions to test for mediation effects by examining associations between cortisol-related regional biomarkers and cognitive measures, at P < 0.05. To reduce the number of comparisons and therefore the potential for Type I error, this analysis was limited to cognitive measures demonstrating significant sex modification effects in their relationship with cortisol levels.
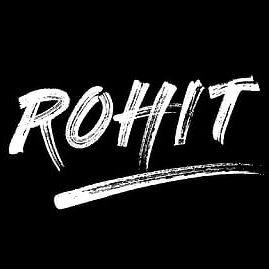
Rohit Malhotra is a medical expert and health journalist who offers evidence-based advice on fitness, nutrition, and mental well-being. His articles aim to help readers lead healthier lives.